Pragmatic clinical trials: Ideal in theory, difficult in reality
Sponsors are keenly aware of the challenges of standing up pragmatic clinical trials. From defining the right cohort of patients to efficiently collecting and analyzing real-time data from various sites of care, there are a lot of moving pieces to juggle.
Still, sponsors are aware of the growing value these types of clinical trials promise. Prospective collection of real-world data (RWD) for both control and intervention arms is an innovative way to generate evidence in support of your product. And the need for real-world evidence (RWE) generated from these data is only growing. Payers, healthcare providers and regulators continue to raise their expectations for quality data to support their decision-making.
But you may encounter common roadblocks when trying to execute a successful pragmatic trial. For instance, patient recruitment is a challenge for any type of research study. About one-third of trial sites fail to meet their patient recruitment goals. Finding patients eligible for your clinical study is one feat, not to mention keeping them enrolled and engaged for the duration of the trial.
Plus, pragmatic trials reflect real-world settings, and real-world clinical practice is often messy. And obtaining regulatory-grade RWD from these settings in real time is a tough task. Healthcare providers collect patient data in several ways across many sites of care. And as patients switch jobs, travel, and move, their health changes over time — meaning their personal health data are stored in potentially dozens of different places. Tracking this information throughout the duration of the study is not an easy thing to do.
Once you’ve identified your data sources, you’ve got to sort out data collection to ensure you have the information needed for reporting and analyses. But all those different data systems we just talked about? They all store patient data differently, from the way dates are recorded to how structured fields are reported.
Even after your research team tracks down all these data from all these places, they must evaluate each field and translate the information into the study’s electronic data capture (EDC) system. But when you introduce a human into that data capture and manual transfer process, you also introduce the risk of human error. Mistakes can mean the difference between hitting or missing your study’s completion date and can undermine the confidence in the evidence you spent so much time, money and energy on.
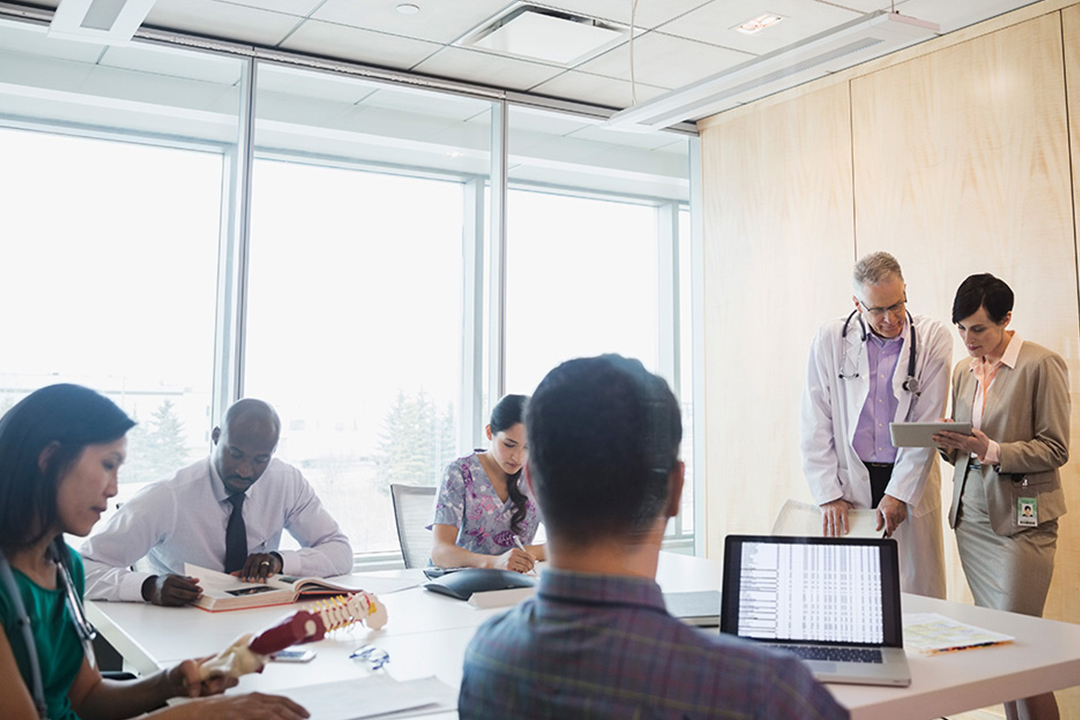