Video
Maximize your real-world data strategy
How might common pitfalls impact your work with real-world data (RWD)? Discover how to make the most of your investments.
- [Eric] A very catchy title of the presentation today, "How Executives Can Help Avoid Common Pitfalls When Working with Real World Data." If nothing else sticks in your mind after today, I'm sure that will be it. Now today's presentation is we are with an organization Optum. We license Real World Data, Optum Life Sciences division specifically. And the reason that we're having this discussion is myself and my colleague Lou, who we'll introduce ourselves more formally in a moment, we have a lot of conversations with life science leaders across the industry. We have probably had in excess of 100, 150 conversations this year, I would say, and in a lot of those conversations, we are having discussions with executives and they're bemoaning the fact that they're not necessarily deriving the value that they would like to derive out of the real-world data assets that they license. And so what this discussion that we'll be having today really represents is the distillation of a lot of those insights. You know, eventually you start to hear a lot of recurrent themes, they repeat, you start to sort of put them into categories and buckets and then we can offer some solutions to those executives to sort of talk through that. So this discussion here is largely about our experience working with those life science leaders. But I will emphasize this probably something in this discussion for anybody who is either working with life science organizations or working with real-world data in another industry of healthcare. So I'd just like to emphasize that point. Okay, couple of tips and tricks here. More on the right hand-side than the left-hand side. Again, we will be polling here. Just a reminder, make sure you hit that submit button. We did a couple of test runs with this with some crew at home, and I kept forgetting to hit the submit button. And again, if your phone screen does go blank open, let the app refresh. No need for anxiety. It'll all be okay. All right, while I'll start out introducing myself, my name is Eric Fontana, vice President of Client Solutions with Optum Life Sciences. I spend a lot of time working with a team, roughly 25 folks. We work very closely with data scientists, analysts, researchers at life science organizations across the country as they derive insight and value from our real-world data assets. So we spend a lot of time pretty deep in conversation, really helping, you know, navigate, understand, you know, the different data substrate that we work with. And so that's primarily what I do with our organization. I'll pass it to Lou.
[Lou] And I'm Lou Brooks. I'm the Senior Vice President of real-world data and Analytics at Optum. My job is really focused on helping clients from strategy perspective. I lead the organization, I lead the data strategy for Optum and what we do with real-world data and providing it to clients for research purposes, as well as engaging various life science organizations in what they want to do with data from that perspective. So welcome everyone. We hope the next 40 minutes or so is informative and we look forward to walking you through it.
[Eric] Okay, we're gonna start out with the poll. We told you a little bit about us. Now it's time for you to tell us a little bit about you. So question number one, what type of organization are you from? Life science, top 30 or non-top 30, provider organization, life science adjacent payer or other. If you can open your answer pad, pound the answer in, we've got 10. Oh wow. Look at those answers rocket up. This is exciting when you do it in real life, Lou.
[Lou] I know we thought, you know, we thought it would be a good idea. Get some engagement. They've been sitting all day listening to people talk, you know.
[Eric] Keep the answers coming. Once everybody answers the question, the results should pop up automatically. Let's see, we've got over 30. Look at that, other. So not a huge life science representation here today, but I think that this should be interesting. And we have a lot of folks in the life science adjacent space too. So welcome to all. Alright, we've got a little bit of a roadmap for today's discussion. Three things that we're gonna go through. First, we're gonna start out going through some top-of-mind concerns for life science executives involving real-world data. And that will be a couple of the things that we talked about from the outset of the discussion today. Those will be some of those challenges that are classically faced. Then we're gonna start to boil those down a little bit more. So we'll go through the organizational challenges for the life science industry when they're actually working with real-world data. Lou's gonna provide us with a lot of his perspective and insight over, you know, 20, 25 years working in this space. And then we'll wrap up with some closing thoughts and recommendations. So we want this to be a relatively solution-oriented discussion to the best degree possible. Anyone know what this video game is? Every step is a potential pitfall. This is very true when working with real-world data. It is, as we often say in Optum, it's no coincidence that gator rhymes with data. I don't say data like data, I normally say data, but in this case it works. So this is gonna be a little bit of a test to see if you get the survey platform is working for you and an opportunity to answer your video game knowledge. What game is this? Mario, Sonic the Hedgehog, Pitfall or Minecraft? The answers are rocketing up the charts here. Nine seconds left. Pitfall. That's fantastic. That means one of two things. You either have played that video game and are really old, like us, or you got the hint on the previous slide. Definitely not Minecraft. My children would be offended by that answer. All right. So let's start out with the discussion here. Two main executive concerns that we wanted to hit on. And again, you know, we mentioned from the outset of the discussion today, Lou and I have a lot of conversations with life science executives as we travel across the course of the year, this year, including, you know, a really big forum where we sat down with some folks in Minneapolis earlier this year and had, you know, some of the prominent life science organizations in the industry sitting with us. And really what we heard were two key challenges. The first is, you'll see on the left-hand side here, how do we maximize the return on investment of our growing real-world data investments? And then the second one really pertaining to playing the role of sponsor in a study for the FDA and ensuring that sponsors can understand and explain real-world data to the FDA. And so this very gonna turn to Lou a little bit. Lou, I think one of the questions I'd like to, or insights I'd like you to provide for the audience here. When we hear about return on investment, we sort of think about where life science organizations are today and where they would like to be in the future. Could you illuminate that situation for us.
[Lou] Yeah, I think, you know, one of the challenges that many organizations have, and I don't know that it's necessarily tied specifically back to data, but data is one of those large investments. Companies are spending millions of dollars on real-world data to support various types of research and analytics within their organization. And there's no formal mechanism for them to measure return on investment. A lot of organizations are counting uses of data or publications or things of that nature. And you know, one of the key questions is really what's the value of an article in JAMA for my business as an example. And organizations are utilizing the data they're getting, but they believe they're getting value out of it. They see it in their engagements with providers and payers, but they haven't yet gotten to a point where they've got a formal process in place to say, yes, I made a $20 million investment in electronic health record data and it's returning to me, you know, 40 million or $50 million in incremental benefit based on all the analytics that we're doing. And a lot of that is driven by some of the challenges that many of these organizations have around how they're structured. You know, do they have a centralized center of excellence or are they fairly siloed? How well is their organization set up to track various types of financial activities? Do the organizations themselves really value and focus on the impact that their research is providing? Or are they primarily focused on the end game, which is just getting the research done itself? And I think many of these firms are slowly working on figuring out how to set up their teams so that they can truly get a better sense of what the value is for each investment, especially as the data market becomes more and more fragmented with more organizations coming out with different sets of data, with other organizations coming out with novel types of data, gets harder and harder to figure out exactly what we're getting from that work.
[Eric] Great, thank you. And on the flip side of the equation, challenge number two that we talked about ensuring that sponsors understand and can explain real-world data to the FDA. Question for you is, do you perceive that primarily as a knowledge challenge for these organizations?
[Lou] Now, it's more a, it's more of an evidence challenge, frankly. It's more about the providence of the data. How does the data get curated? How is it built? What are the various components that go into that particular solution? Because as the FDA is looking to make decisions or determinations based on the use of that real-world data, they're keenly aware of the pitfalls that exist. We've talked about and heard throughout this conference about issues with health equity and diversity and things like clinical trial, real-world data suffers from the same thing. We know a lot about the people that engage with healthcare. We don't know a lot about the people that don't. And even with the people that do, there are various types of limitations to that data. And being able to understand that and educate those that are going to consume the final results become extremely important from that perspective. Especially when you compare it to the current standard, which is randomized clinical trial data.
[Eric] So a little poll here. Which of the following best describes your organization when it comes to measuring ROI from real-world data? Now I understand, you know, we have a limited representation of life science here today, but I'm curious for folks who actually do work with real-world data or have worked with real-world data, whether you have a perspective on this. So the first here we have well developed empirical methods for calculating the ROI. Second in blue we measure value anecdotally, no formal methods. In the yellow, we mostly just track utilization as a proxy and in the green, bottom right, we don't know. Curious to hear folks perspective or even if you would like to just vote what you think might be the case could be interesting as well. Let's see. We've got nearly 10 seconds left to answer. All right, let's see what we've got. Okay. Pretty even split loop between anecdotal measurement and then not knowing the true value, Lou does that surprise you at all?
[Lou] No, that's consistent. I mean that's consistent with the conversations we've had. you know, that's where we're at today. You know, unlike let's say a promotional spend or some other type of intervention where there is a defined mechanism for evaluating the impact it's had on outcomes or on care for that particular set of individuals. You know, people don't necessarily think about data in that same way. They're not necessarily set up to evaluate whether or not it's a positive return or it's just a useful foundational element for their business.
[Eric] Yeah. Well I wanna point out the lone wolf that does have well developed empirical methods. Please come and talk to us at the end of the presentation 'cause we'd love to hear your story. Alright, so let's get into some of the fundamental concerns here. And there are five that we've basically boiled down. There's probably, you know, a few more that would float around here. But categorically this is how we think about it. And again, this is based on, you know, a hundred plus discussions this year. More often than we'd like, we see life science organizations not exploring the full range of possibilities for the data. Fully optimizing data talent for success, not adequately considering privacy risks when working with real-world data. Not appreciating why real-world data doesn't always contain the elements that researchers want, and not silo-busting within the organization to optimize licensing, strategy and impact. So these are the categories we're gonna walk through each in turn. And another poll question coming up here. This is a multiple choice. Which are the following are a problem for your organization when it comes to real-world data? We'd like to get a little bit of insight to the degree that we can here. And again, we have a minute to answer, I'll read them out. The red one here is using the data to its maximum potential. Blue is fully optimizing data talent for success. Yellow is adequately considering privacy risks when working with real-world data. Green is developing and maintaining institutional knowledge around real-world data. Teal, bottom left is the organizational silos and purple is none of these, but we have some other real-world data challenges.
[Lou] In this particular case, there is no one right answer. So if you've got more than one you can check multiple boxes and we'll ultimately tabulate those results.
[Eric] 10 Seconds left. 3, 2, 1. And let's have a look. I feel like I'm hosting a game show Lou. Using the data to its maximum potential. Surprising to see that as the far and away number one result and then optimizing data talent for success. That opens some doors to some interesting narratives that we'll see here. But Lou, what are you, what's your takeaway from these results here?
[Lou] You know, it's not surprising. I think, you know, one of the, you know, one of the challenges as we think about those first two boxes, they kind of go hand in hand.
[Eric] Yeah.
[Lou] I mean one of the things that we see in healthcare, we see a large migration of talent in the data science space from consumer packaged goods, tech, many major firms are looking for that talent. The piece that they lack is the knowledge of the healthcare space.
[Eric] Yeah.
[Lou] it's not an easy transition to make. It's a transition I made 27 years ago from telecom into healthcare. It takes a little bit of time to get your feet under you. So you know, with a staff that may not have all of that experience it can be difficult to get to the full value of the data because we don't know exactly what we need to do with it.
[Eric] Absolutely. And that brings us onto our first challenge. So when can, and should we use real-world data to generate evidence? Look, the slide here that we have illustrates, you know, four sort of categorical areas. We can use it for decision making obviously whether that be, you know, clinical efficacy for specific populations, we can use it to innovate at our organizations. That might be things like establishing some unmet needs or drug discovery. We could use it for patent identification around things like tracking or responding to spikes in utilization or cost. And of course establishing value is a really big one that we have a lot of conversations with our clients with that could be demonstrating the value of new drug indications or determining safety and efficacy of a new drug or device. But I think what's far more interesting, our partners that we work with at organization Datavant, they actually conducted a survey a little bit earlier this year and it was a really interesting survey I thought. It looked at the executive perspective from a number of the top 30 pharmaceutical companies in the country. And it asked them about their perspective on what they thought they could accomplish with real-world data versus how well they thought they were leveraging that real-world data today. And you can see almost in every category across the bottom of screen here, whether that be supporting regulatory submissions or reducing cost of clinical trials or optimizing patient support programs and everything in between, just an enormous gap between what the potential for this data is and how they felt they were utilizing it. And Lou, when you look at data like this, what do you think?
[Lou] I think ideally it offers a world of opportunity for us to continue to grow and learn. Certainly as you look at the desire to be in a certain spot and where people believe we are at today, it gives us a lot of runway to work with, in terms of how we collectively get better at deploying real-world data assets across the organization.
[Eric] Absolutely. Now problem number two or challenge number two was actually one that the group here highlighted fairly significantly. And this is the failure to optimize talent. Now this is a extraordinarily deep discussion. In fact, you know, most of the conversations we've had with our life science leaders, this can go on for in our experience hours talking about this because it is very multifaceted. But the three big buckets of areas that we largely look at will be things like hiring. So challenge is not just around hiring the right talent, but then how do we actually go about ensuring that they have the requisite knowledge coming in to be able to hit the ground running to a degree. And where we have that, or perhaps don't have necessarily that ability to do that, the training and support for those folks as well too. Because we work a lot with organizations helping them orient, navigate, understand what this data substrate is that they're licensing and developing that institutional knowledge is a really critical thing. But perhaps, and Lou may even argue, that this third one is the most fundamental, is the leadership component. Now a lot of this leadership component comes down to, you know, setting a vision for the organization, making sure that we've planned appropriately so that the projects we wanted to work on actually have the right data assets to be able to support those. But Lou, could you maybe offer some comment on that leadership component? 'Cause I know you and I talk about this a lot.
[Lou] I think, you know, that's, you need vision on what you wanna do and where you wanna go from a data perspective. And I think that's part and parcel of the challenge in maximizing the value of real-world data. You know, you have to sit down and lay out the plan, why do I want this data? What are we going to do with it? How are we going to consume it? How are we going to use it? What insights are we going to generate? And that vision is extremely important. It also lays out the roadmap for how you're gonna get there. What are the types of people that you need to hire. One of the things that we see quite frequently in limitations in leveraging the data is got very strong data science presence, maybe lacking on the clinical expertise. So having individuals that are really good in statistics and computer science and mathematics is great from a pure modeling standpoint. But you need that context from a clinical perspective to help guide some of that model development. And if you don't have that balance set up quite right and you don't have that vision, you ultimately end up with a suboptimal team who struggles to really drive the data where it needs to go.
[Eric] Absolutely. I couldn't agree more. Okay, A poll question for you here folks. Which of the following is the biggest talent challenge for your organization when it comes to real-world data? Let's have a look. Leadership, hiring the right people, centralized acquisition, enablement and access? And that includes compliance, training and institutional knowledge or none of the above? We are crushing it in all areas. 40 seconds. Let's see where we're at with this. 30 seconds left. 15. Looks like the answers have stopped. I wonder if I can proceed it without breaking it.
[Lou] Try it.
[Eric] Try it.
[Lou] Or don't try it.
[Eric] Or don't try it.
[Lou] We didn't test that.
[Eric] we yeah, we did not test.
[Lou] Wanna skip that?
[Eric] I will admit. Yeah, there we go.
[Lou] Scattered pretty much all over the place.
[Eric] Yeah, it is. It really is. Maybe the training and institutional knowledge is perhaps not surprisingly one of the big things that we obviously work with clients a lot on, right?
[Lou] Well, it's one of the, yeah, it's one of the things that we struggled with early on.
[Eric] Yeah.
[Lou] I mean about 10 years ago we first brought electronic health record data to the market and we struggled to get organizations to appropriately utilize that data relative to the experience they had, using claims data, different systems, different data sets, different variables.
[Eric] Yep.
[Lou] Claims methods, as you know, don't translate directly all the time to electronic health record data. And it takes a bit of time to build that knowledge and focus on how to look at that data a little differently. So no, I'm not surprised that that one pops up to the top, given some of those challenges.
[Eric] Fantastic. Little bit of a leadership challenge there and hiring the right people. But that's a pretty even spread. Okay, we'll move on to challenge number three here. We're gonna talk now about patient privacy a little bit. I think this graph, we can move through this one relatively quickly, but you know, everybody here is aware of HIPAA, right? This here is a chart highlighting the Office of Civil Rights, which is a part of the Department of Health and Human Services, HIPAA penalties going back over from 2008 through to 2022. 2022 obviously hasn't been finalized. So we don't have the full set of data, but I think you can all agree the trend line is going up. And this is probably to be expected, just given the relative explosion we've seen in data utilization in that period of time, right? But one of the interesting points about this is that almost all of the violations, if you go through them qualitatively, are not willful misuse, it's carelessness, lack of compliance with standards, that type of thing. Not ensuring or safeguarding the data appropriately, but not really any cases really of willful misuse. That picture gets a little bit different when you move over to this next category, which is talking about the types of fines that the FTC or even the European Union gets involved in. And here we start to talk about some, you know, bigger, more well known high profile companies. But one of the things that is undoubtedly true is that regulatory organizations across the board are paying much, much more attention now to the fact that data is being used and employed and working really hard to safeguard patient and personal privacy in all cases. And where the rubber really hits the road on this in real-world data, at least in one of the situations that Lou and I work most closely with clients on, is when we get into data linking and that is taking particularly a de-identified asset and then linking it with another data asset to try to derive specific insight. And Lou, I wanted to pick your brains a little bit on this concept 'cause I know you and I have some very animated conversations around data linking and the potential risks associated with it. But talk to me a little bit about the common phrase that we often hear is why can't we link these two data assets together?
[Lou] Yeah, this is the one thing that keeps me up quite frequently. I spend a fair amount of time on this. Patient privacy obviously is at the forefront of all we do from a research perspective. You know, we want to be able to use the data to advance the insights that we generate, but we need to make sure that it remains confidential from that standpoint. And I think that the explosion of tokenization and the desire and need to build more bespoke data sets for very specific uses, whether that's for clinical development purposes, for answering specific questions, comparative effectiveness analyses, whatever they need has created this scenario where, okay, we think that two de-identified data sets added together equals another de-identified data set, which isn't true. It can be, but you need to have the discipline to ensure that you are actually checking to make sure that that data remains de-identified, linking is here, it's going to continue from that particular standpoint. And it is a good thing to enable new ways of joining data and conducting analytics. But you have to be very careful and, you know, what we will recommend is making sure that you've got an organization has a compliance team that is sitting on top of what their researchers want to do with various data assets. Because as Eric indicated, it never is intentional. It's typically accidental. It's more about not knowing what you should or should not do. So if anything, we recommend that you ask yourself one question when you're going to link data, should I do this? And is it still de-identified? And if you can answer those two questions, then you can move forward. And if you can't, then you should make sure that you get the answers to those questions before you move forward. Because the risk of this is high. And the more that we try to build these bespoke data sets, it's only going to grow up. And it's not worth the reputational risk for an organization, the financial penalties from an organization and, you know, taking an extra day to a week to make sure things are where they need to be is worth getting that taken care of without having to deal with the repercussions.
[Eric] Like Lou always says, you don't wanna end up on the front page of the Wall Street Journal for the wrong reason. Okay. Which best describes how your organization thinks about data linking with real-world data? So let's have a look here. We do it often. We've done it once or twice. We haven't done it but interested or we haven't done it and we're unlikely to.
[Lou] And as we think about linking, I mean that is, I mean that is the future. I mean even an organization as large as Optum and other organizations that are working with, you know, building out real-world data for research purposes, no one's ever going to have the complete set of data that an organization may want. Whether it's genomic information or images or lab results, whatever the case may be. You know, we build these assets and we're able to do a great deal with them, but there's always a need for something specific. Maybe it's a specific biomarker or specific genetic test that an individual lab has that you want to be able to join with general phenotypic data to conduct some type of analysis. And so we just need to make sure we've got good guide rails around it.
[Eric] Yeah, and I think the other thing, the other point that's worth making as well too is we see increasing fragmentation in healthcare. You've probably all read the trends about the increasing outpatient shift and the move to hospital at home and, you know, more ambulatory sort of controlling referral channels, particularly for those in the IDN world. That's a real and material business threat. But as the ecosystem of healthcare delivery starts to fragment, we start to see more and more of the data fragmentation that goes along with it. So interestingly here we have the vast majority saying, we haven't done it and unlikely to, but you know, some folks saying they've done it once or twice. A lot of experience actually here, some folks doing it often as well. Cool. Alright. True or false here we're gonna test you here. Linking two de-identified assets together for greater insight always results in a de-identified data set. True or false? The answers are going up quickly. Five seconds here. We're gonna test to see what's in those coffee cups of yours late on this day two afternoon.
[Lou] So there's at least two people that weren't listening. That's just the way. That's the end. That's it.
[Eric] That's just the way it goes.
[Lou] We can move on.
[Eric] Yeah.
[Lou] There we go. All right, for those two, you can come talk to us later as well.
[Eric] That's okay. We forgive you. These data don't contain the elements we'd expect. This next challenge is the one that we talked about with organizations not really understanding the data and for our experience, there's really two sort of key challenges that we see here. The first, physicians and patients don't often behave as you would expect. You've probably sat here and listened to a number of presentations over the course of the last day or so. You've probably been to a number of other conferences talking about, you know, socioeconomics, you know, behavioral economics, clinical decision making and clinical variation. They're all very common themes that you'll hear about in healthcare. But there is a lot that feeds into why an organization might look at a data asset and see certain types of patients that they would expect to receive a particular course of therapy or a surgery not receiving that. So that's point number one. Point number two is that medicine typically isn't practiced like a clinical trial. And so we all know that the data scientists that we often work with are sometimes expecting beautifully curated data like they would see from a clinical trial with all of the same outputs, all of the same sort of, you know, might be patient reported outcomes, it might be certain types of, you know, findings that they're tracking depending on, you know, what therapeutic area they're investigating. So Lou, maybe you can talk a little bit here about these, the perception that, you know, real-world data sometimes doesn't contain the elements we'd expect and how organizations work around that.
[Lou] My aunt's a physician, she's been a physician for what, almost 50 years. She's always told me medicine is messy, right? You know, you work to figure out what is going to meet the needs of that individual patient and you know, you move with it and sometimes that means you pivot if something's not working, but it's not cut and dry. Every patient is individualized in the way that she practices medicine and has over the four decades of her career. And I think that that's the critical element that we always have to remember here. The way to mitigate this particular risk is twofold. Mentioned it earlier, clinical resources on your team so that you have that perspective. And number two is make sure that you are working with a broad-enough set of data that's the most extremely important thing that we can talk about. The diversity and representativeness of the data asset that you are leveraging will mitigate the situations where you see the unexpected. Because it's going to happen. Whether it be because a patient has a particular contraindication with a therapy or a particular side effect that resulted in that therapy being stopped. They're on a different therapy that countries indicated for that therapy, whatever the case may be, there are going to be differences in the data and it's not a bad thing. In many cases you can learn something from what you're not expecting to see, not necessarily what you expect to see.
[Eric] I think that's right. Absolutely right. So our last factor here is talking a little bit about, and this might even be the biggest factor that we're talking about because it does weave a lot of the themes that we've touched on today together. And this is the problematic organizational silos that we often observe across life science organizations. And so here we've bucketed out five of the types of things that we will often see. When we mean organizational silos, classically what we're talking about is different departments within a larger organization that don't necessarily work together in a cohesive manner to ensure that the data is procured, stored, and made available to the rest of the organization in a way that everyone can access it in a relatively democratic fashion. So we would see things like not having an organization-wide real-world data strategy and planning department. We would see decentralized purchasing and lack of coordination. We would see limitations for internal access. So we may acquire a data asset but we might not make it available to everyone who needs it. We would store that sometimes in a decentralized fashion. So there might be some cases where we have duplicate licensing because there's a data asset that's acquired by an organization and there's another team who would like to use it and don't know they already have access to it. And then no mechanism to grow and develop that institutional knowledge. And these are, I would say classically some of the biggest barriers that we see that drive a lot of the other factors we've talked about today. So Lou, I know that we have sat down and had a lot of sort of extensive conversations this year about a framework for this organizational silo busting. And we've got sort of six steps here, but I'd love it if you could just walk the group today through what high performing data organizations share in terms-
[Lou] Sure.
[Eric] of common characteristics.
[Lou] Sure, I think the first is that they've got some presence from a central organizational standpoint, chief data officer or other subject matter expert that's helping to guide and lay out that strategy. The second is they formalize a strategy on what they wanna do with RWD and RWE and they make that available to the entire organization. and then they centralize all of their data procurement and enablement functions within that group. Build that out, start small, you know, it's not gonna be built overnight, neither was Rome. So we ultimately need to work at this slowly but steadily and invest in the right infrastructure, take one, two data assets as a starting point and then build from there. And as you scale that, and as you can demonstrate the success and the cost savings and the time savings associated with that centralization, you can continue to get the rest of the teams built into that and streamline how you grow your overall use of data.
[Eric] Great. No, it's easy to boil down into a slide, but it certainly requires, as we mentioned before, the right leadership. So a question, which of the following silo indicators have you observed at your organization? You could put here within the poll, something that you've observed in organizations that you've worked with. No organization wide real-world data strategy and planning, decentralized purchasing and lack of coordination. Limited internal access, decentralized storing or no mechanism to grow institutional knowledge. And you can do a multiple selection in this case. You don't have to pick just one.
[Lou] We've only got.
[Eric] We are at nearly two minutes and we've got 30 seconds left here in the poll. We'll be good. Any more answers?
[Lou] I forgot to compliment you on your ability to track down such nice pictures.
[Eric] It was stock image.
[Lou] Yeah. [Eric] Yeah.
[Lou] I'll have to.
[Eric] Well only the best-
[Lou] We'll have to talk to marketing about that.
[Eric] Only the best.
[Lou] Very nice job. Making sure we have appropriate-
[Eric] Well, you know, I am a farmboy, right?
[Lou] I know.
[Eric] Yeah.
[Lou] Those days in the Australian outback.
[Eric] There we go. No organizational real-world data strategy and planning, number one, that I think pretty much aligns with what we see.
[Lou] Yeah.
[Eric] Okay. So very quickly setting your organization up for success. We have provided here some sample questions that you can ask both your organization and then also potential data partners that you may wanna work with. And we will, we could go through this, we could spend a significant amount of time sort of talking through this. However, we have provided for folks taking a picture of the screen right now. There is a hard copy outside for you. You can pick it up and we'll be happy to provide that for you. So there should be at the desk on the outside, maybe at the table, at the back somewhere. But I'm not exactly sure where it is. But it's-
[Lou] should be out on our Optum table.
[Eric] Should be on the Optum table, hopefully. So that will be there. Quick poll question here. Actually you know what? Let's see if we can skip through this one. If I could only solve one real-world data problem at my organization, I would use data for a broader range of analyses and users for greater impact, develop better methods for understanding impact, value and ROI, facilitate more data linking, help my researchers be smarter about understanding the details, breaking organizational silos or having more seamless planning, budgeting and funding. This one is the interesting one, by the way. This is the one I really wanted the answer to today. It forces us to take our classic a hundred Pennies and push them all into one specific area. So I'll be curious to see where the group spills out.
[Lou] Okay.
[Eric] Five seconds. Any more answers? Going, going, gone. Used data for a broader range of analysis.
[Lou] Greater impact, Greater impact with the answer to the other question earlier.
[Eric] Yeah.
[Lou] Maximizing value.
[Eric] Like it, like it. Okay. One last little piece that we'll want to end up on here and I'll let Lou in a moment take the reins on this one. But just some final thoughts on maximizing value from real-world data. The concept of a center of excellence comes up a lot when we talk to organizations. And different organizations have different visions and ideas for what a center of excellence looks like. From our vantage point when working with organizations in the life science space, we largely see these three categories as being very important. Lou, maybe you can illuminate why we've sort of gone and chosen these.
[Lou] Yeah. I think the general theme here is you need to be able to support your teams to gain the maximum return on the investments you're making with data. So you need sherpas or expert guides that can help train new resources, can answer questions on those data assets, can really help newer researchers gain value from the data more quickly. Education and training is extremely important. Limiting the amount of time a researcher has to sit figuring out what to do with the data. Minimizing that as much as you can is extremely important. And I think the last piece is the hardest to do. We struggle with it sometimes at Optum as well, which is self-service resources, right? It's really easy to say, hey, Eric is the expert. Go talk to Eric. Well Eric's busy. Eric might have a line out the door of his office. Well, you used to when you were in the office-
[Eric] Had an office, yeah.
[Lou] Now it's a line on Zoom waiting to get in. So building out the FAQs and code libraries and other types of self-service solutions digitally, extremely important to enable people to be more efficient and more effective in working with the data and not being reliant upon someone to get around to answering their questions. So I think, you know, as you think about setting up an organization that's going to engage and work with data, making sure you've got these three components very important to maximize that return on investment.
[Eric] Fantastic. Well, thank you everyone for your attention today. We've run to the end of time. I hope you learned a little bit about life science and its interaction with real-world data. And if there are any questions or you wanna come and have a chat to us post presentation, please feel free to mosey on up. Thank you and have a great rest of the conference.
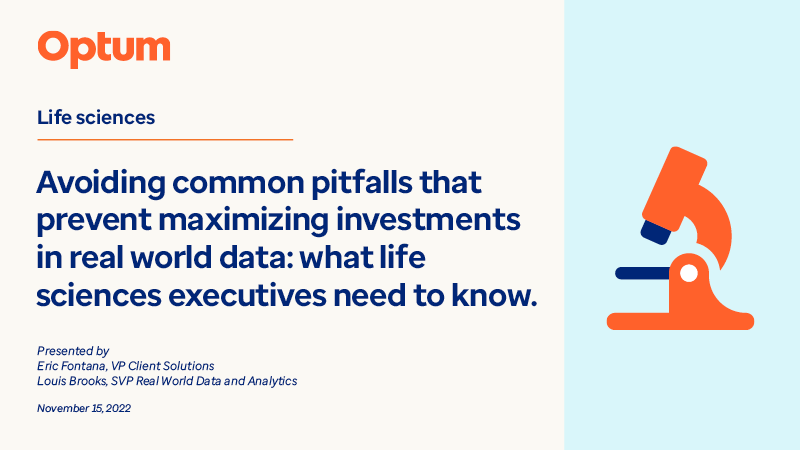
What life sciences leaders need to know to enhance RWD value
Hear Optum experts Lou Brooks and Eric Fontana break down common missteps when using RWD and offer practical strategies to overcome them. Presented at the 2022 STAT Summit.
Related healthcare insights
Article
Understand how routine clinical practice impacts information captured in real-world data (RWD).
Article
Providers collect data about patients every day. But what should they be considering — and doing — to put their clinical data to good use?
Article
An innovative program involving patient awareness and provider outreach improves patient use of lipid-lowering therapies.