Webinar
The increasing potential of genomic data
Hear from industry experts during this Endpoints News webinar on the ever-increasing importance of clinicogenomic data.
The increasing potential of genomic data
[Eze] - Thank you very much for joining us today, for a very dynamic conversation, on the relevance of drug development, in terms of clinical genomics and novel types of real-world evidence. And so, once again, my name Eze, and I'm currently part of the Optum Life Sciences team, supporting the commercialization of various new products to our Life Sciences customers, but also, really with attention, as well as, any focus on relevant stakeholders, across the healthcare value chain. And so, before we begin introducing our panel, I would like to just thank Endpoints and their webinar series, for being able to bring us together, in this particular medium, for this very impactful dialogue. Also, for Novartis, as well as, our corresponding panelist, from Guardant Health. In terms of just a few housekeeping items, before we delve into the actual conversation, if you have any questions during today's webinar, our panelists have reserved some time for questions at the end of the webinar, and so please hit the Q and A button, at the bottom of your screen as soon as you think of your relevant question, and we're happy to address it. Either live during the dialogue, or as a follow up after the conversation. For those of you that may be interested in referring back to the webinar and the audio, after today's discussion, it will be available on demand tomorrow, so you can rewatch it or share it with your colleagues. And so, with that being said, just wanted to introduce our panel for today's discussion. Leading off is Nuray Yurt or Dr. Nuray Yurt, sorry, Nuray, who's a PhD and the Global Head of Artificial Intelligence, for Novartis Pharma. In addition, my colleague Ashley Brenton, who's also a PhD, with a particular emphasis on genomics, is also participating, and she's a Vice President of Real-World Evidence and Genomics for Optum Life Sciences. Last, but certainly not least, is Naveen Kumar, who's the founder and head of GuardantINFORM. And so, we have brought together this esteemed panel, to support a very impactful discussion, on the relevance of clinicogenomics, across the Life Sciences from discovery, through R&D and through commercialization. And so, the first topic that we would like to address, was a key point of interest for this industry, Life Sciences, in particular, at the onset of this year, which is, essentially, how the FDA was providing guidance to, really healthcare stakeholders, and certainly to pharmaceutical entities and, broadly, Life Sciences, on how to leverage real-world data and evidence in the regulatory submissions, as well as, in their general evaluation of drugs and biological products. I thought this was a really interesting topic, to kick off our conversation because it really, kind of, spurs off and builds off of the 21st Century Cures Act, which was the first, kind of regulatory filing, from my perspective at least, that does support formal guidance, on how we leverage RWD - as well as E - in the regulatory decision making process. And so, I wanted just to begin by touching- We're asking Nuray from Novartis, to provide her guidance, as well as her perspective, on how, kind of, critical and relevant this guidance from the FDA was, in supporting how we, as data scientists and those that leverage RWE, moving forward.
[Nuray] - Yeah, thank you. Thank you, Eze. So, it's great to be part of this panel and having a discussion on this. I think it's actually very exciting. I mean, to me, this was like super good, great news, that FDA actually now cares about data, right? Like, so we all say that, okay, yeah, of course the pharmaceuticals is a data driven industry. It's all about the data. But then the data, the description of data, was always limited to clinical trials, right? Like, so it was always limited to the data that's actually captured from the patients. And with this actually guidance now, FDA kind of confirms the acceptance, of all the data that's out there, which is being captured, to its value. So, for those who have actually looked at the documentation and the guidance, you will see it's still a very, very high level guidance, you know? So, it's basically, now we're saying, okay, yeah, EMR, EHR data is accepted, genomics data is accepted, you know, claims data is accepted, medical claims data is accepted, but there's really not much depth into it. I think, this is a great step, you know, right? But I think we still have to talk about, eventually, quality of the data, which will actually improve the whole industry, on its own. I mean, as you can imagine, data scientists, like us, who have been in this world for a while, we do care about, not only where the data comes from, but the quality of data, how it was captured. And that's why, sometimes, you see the discrepancy between the trials and the real world because of the data selection bias, all of it, right? Like, I think that data scientists, can easily explain this gap. So, to me, it's actually super exciting, that we're actually going into and starting a new era, from the FDA point of view, but I think there's still a lot of discussions and enhancements and a little bit more depth to it, that needs to be done through the FDA. Which, most probable, will likely also start, with some more data science experts to be employed by FDA. And then, so it kind of, expands from there.
[Ashley] - I thought it was particularly interesting how -
[Eze] - Thank you so much, Nuray. And I think -
[Ashley] - I think we have a freeze. So, I was just going to say, I thought it was really interesting, how they called out the difference, between real-world evidence and real-world data. And Nuray, that really points to what you are saying about the quality of the data because that determines the quality and the utility of the evidence.
[Eze] - Thank you so much, Ashley and someone... About how the guidance from the FDA, in terms of how we leverage RWE, impacts cancer diagnostic manufacturers, if not institutions, such as Guardant, as well as your broader, if you will, industry.
[Naveen] - Yeah, sure. Absolutely. And so, first of all, I do agree that, you know, a lot of work needs to be done, but I think it's worth, you know, commending FDA and taking a leadership position on this. You know, I think the broad framework, that they put out, was three years ago, and I think we've seen a lot of what they said come into practice since then. So, you know, worth, you know, citing some progress here, before diving into, sort of, what can be changed and modified. From a diagnostics perspective, you know, in some ways, we've sort of trailed the therapeutics sector, in terms of, the use of real-world evidence, but nonetheless, you know, there's no reason why we couldn't leverage the same methodologies, the same frameworks and the same techniques, in terms of data generation and evidence application, for diagnostics regulatory purposes. So, I think that is something we'll certainly hear more about, over the coming years. And I'm, you know, I, for one, and certainly being at Guardant Health and working in the real-world evidence space, really, am looking forward to that.
[Eze] - Thank you so much, Naveen. And so, just switching gears, to a very unique example, of how impactful clinicogenomics, or really genomic therapy developments, can truly support how we can impact patients, through innovative medicines, I wanted to just call out, at a high level, this article from the New York Times, that addresses a unique, smaller scale trial, where 18 patients, in the rectal cancer space, all experience a fantastic outcome, which was remission of their latter stage rectal cancer. And so, this is certainly, a key milestone, from my perspective, on how genomic therapy, can really just support and catapult the industry to the next frontier, in terms of how we can serve and ultimately deliver value to our patients and stakeholders. But would love to hear Ashley's thoughts, on, kind of, how notable this milestone is, in terms of drug developments, from a clinicogenomics perspective.
[Ashley] - Thank you, Eze. This was such an exciting result. This is a study that came out of MSK, like Eze said, it was a small cohort, but patients with rectal cancer, advanced stage rectal cancer, these patients received a targeted therapy, based on their biomarker profile and the biomarker profile of their tumor. And without surgery, without radiation, and without chemotherapy, there was a complete remission of their cancer. So, this means that physical tumors completely disappeared. And this is so exciting because it really delivers, on the promise of precision medicine. This is what we've been working on for so long. And I think it's interesting too, while this is a controversial idea in oncology, but it introduces the idea of a cure. Can we cure cancer with these targeted therapies? So, really exciting. I'm looking forward to following this in the coming years and also, the new development and new work in this space.
[Eze] - Thank you so much, Ashley. And so Naveen, just given the relevance of clinicogenomics and data, if you will, that is an exhaust from the operations, of such innovative companies like Guardant Health. I would be curious to hear your perspective, on outcomes like this, in the real world, in terms of how innovative therapies, can support very complex patients, such as, those with late stage rectal cancer?
[Naveen] - Yeah, absolutely. So, first, you know, there are a few articles, maybe a year, that, you know, working in the oncology space, that you get sent, like, a hundred times that day. And this was definitely one of them, including from, you know, friends and family and everyone else. So, it's always exciting when that happens. So, this, sort of, told me two things, which I feel like we already knew. But it was really good to, sort of, see it, sort of played out, with this sort of data. One, is that biomarkers work, right? So, in this case, this was an entirely, biomarker selected population, with patients with mismatch repair. And, you know, that was a big piece of, I think, why we saw this, sort of, tremendous outcomes here. And in then the second, is that, you know, catching cancer earlier, so prior to the metastatic progression, it helps, right? So, this would be, sort of, somewhat tough to pull off, potentially, in the metastatic setting, given the, sort of, late stage nature of that disease. And so, from a, you know, Guardant perspective and a data perspective, you know, that's exactly, you know, what we try to do. So, starting the late stage space and moving earlier, we're hopeful, that some of what we've learned, in the metastatic cancer setting, regarding, you know, understanding biomarkers and that their association with clinical response, we can start applying into the earlier stage setting, with, you know, even more of an impact, you know, very much on trend for us. And it just, the other thing I'll note here, is that this seems to be, you know, one example, but, you know, this is hard, right? So, what we've seen with immuno-oncology therapy so far, is that they do work and they work for a subset of patients. And then, you know, I think everybody, in the industry at the moment, is trying to understand why. And, you know, circling back to what this, you know, seminar is about here, is, you know, the application of real-world evidence there, I think, is where we are going to, you know, find those signals, so that they can be interrogated further, at least. And, really, you know, better characterize what's happening biologically, for these patients. And I don't wanna skip ahead, but I mean, I think one of the things I'm really excited about, is the, sort of, diversity of data, that we can now bring to bear, you know, beyond, you know, just the clinical piece, but, you know, including the genomics and others, to really, you know, do a deep characterization of what's going on and hopefully, you know, make these therapies work better for everybody.
[Ashley] - I think that's a really good point, Naveen. I really like how, you know, studies like this, demonstrate how important that entire patient journey is. And when we only have pieces of data or pieces of the story, which is what we've treated biomarker data. This really speaks to how powerful it is, when we have all of those different pieces of information and we can see what led up to the diagnosis and what's happening throughout that patient journey.
[Nuray] - And one thing that I wanna add- Yeah, and one thing that I wanna add, Ashley, I think you've touched a great point, is also, the way that you approach things, being very innovative, right? Like, I mean, as much as oncology is an area that we innovate a lot, we're still very traditional, in certain ways that we actually approach things, right? And people are scared, when we deviate from the traditional, what's gonna happen. And that's actually why, some of these data elements, hasn't been accepted, as widely as before. And I think this trial itself, you know, the way that it was done, the way that it was set up, the way that it was actually approached, to me, is also kind of, now the innovation, being more the norm rather than like, you know, a bit more redlined innovation, right? Like, so it's not like that. So, I think it's actually a great example, just because of that, as well.
[Eze] - Thank you so much, to each panelists. And so, to Nuray's point, on the topic of innovation, this is kind of just, a question, broadly, to all panel members. I'm curious what types of diagnostic, or molecular testing, protocols or innovations, do you find interesting? Whether it's from competitive intelligence standpoint, to not reveal too much information, from Naveen's perspective. Certainly from the pharmaceuticals perspective, if you can comment, Nuray. And certainly, as a provider or supplier, to the Life Sciences, we'd love to hear Ashley's thoughts, on other different types of molecular diagnostic tests, which may be compelling sources of RWE, moving forward.
[Naveen] - I'm incredibly biased on this one, so I'll let my fellow panelists talk.
[Nuray] - You're so good, Naveen, you could have... You know, so for me, I mean, I think, from every aspect, it's very interesting. But, over the years that I've been using a lot of the molecular testing data, to either, you know, to understand the forecast, to understand the patient utilization, to understand- What amazes me, is actually still, there's still an adaption curve, that needs to happen, which is amazing. Most of the time we actually see prescriptions or chemos, like a lot of the treatment patterns happen, before the results of the tests. So, we can observe that in real life, which is, I think there's an element of the test results, being not as expanded. So, I think there's a great opportunity, for a lot of the companies out there, to expedite some of these results coming much faster. You know, biopsies or basically the testing orders, like the claims that comes through with it, but also that there is still, I think, a little bit more timeline skepticism, that delay of the testing, will delay the disease treatment. So, I think that is still the concept, in doctors' minds. So, everybody's rushing, to actually get to the treatment, before even the proper testing being done. To me, that's actually super interesting to observe. It's getting better and better, with the new generation of doctors and with the new generation of the testing, becoming a bit more norm. But to me, that's actually super interesting to observe, because we have, I mean, as Novartis has a lot of drugs, with targeted therapy, with mutations, and it's amazing, you know, it's amazing, to kind of, see this still not being the norm, in terms of like, the tests and then the waiting for it and then the results coming, that longitudinal aspect, which is super interesting for me.
[Naveen] - Yeah. And sure, I'll chime in here. So, I think one, the gap in testing and complete genomic profiling, in many cases, is one that is unfortunate, but real. And I think this is something that, you know, we as a society, really, is something we really need to, potentially, address here. I think, you know, better diagnostics, equals better therapeutics. And so, I think, you know, in many ways, you know, biopharma companies and diagnostics companies, and I would say payers too, because I think the health economics studies, that have been, you know, published and out there, demonstrate that, you know, better management of disease, is just better, better for everybody. And so, that's something I think, we as a group, you know, really need to sort of rally around. And I think that's where, you know, our collective, sort of, data sets, can help, kind of, tell that story and more importantly, I think identify solutions. It's not, you know, I don't know that there's gonna be a silver bullet, but it's really, you know, it's the kind of messy things, like changing physician behavior, right? Which isn't always easy and making sure to align incentives. So, that's sort of the macro picture. I think we all have some work to do there. From a data perspective, the thing that excites me most, about Guardant and, kind of, the liquid biopsy, versus traditional, is the longitudinality of the data. And what I mean by that is, you know, especially in this case for example, you know, we're able to look at patients genomic profile, of pre-imposed treatment, and it's sort of long been understood that, you know, tumors tend to evolve, under the course of therapy. And the question is, why and how and how do we, you know, better create models, to predict those things? And that, historically, has been a data start problem. And so, the thing that was most compelling for me, at Guardant, was the ability to actually characterize, that sort of, you know, molecular evolution or progression of the tumor, you know, at scale, using real-world data. So, from my perspective, from a data perspective, that's what I'm most excited about.
[Ashley] - Right. And coming from the data space, I think it's really exciting to see how the types of data, to which we have access, have evolved. So, for a long time, we had access to limited biomarkers, single variant panels, maybe there was a note in the EMR, maybe not, maybe it was in the unstructured data, maybe we could see that a test was ordered, but it was based on a strange CPT code, that said DNA was extracted and then something happened, right? So, it wasn't very useful. And now we're able to obtain high quality genomic data, to link back to that longitudinal data set. And Naveen, I totally agree. I think it's fascinating to see, in real patient data, how tumors evolve in response to treatment. But also to see, Nuray, to speak to your point, how physician behavior changes, in result, or in response to genetic testing or molecular testing. And I think it also really speaks to the role of the patient now, right? We know that patients are more engaged and more well informed. And when we are talking about, Nuray, you were talking about the demand for treatment, ahead of testing results. I think that, patients certainly, have played a role in that, as well.
[Eze] - Well, thank you so much, to each of our panelists. And so, in terms of this particular topic, what really resonated to me, in Nuray's comments, was her reference to, for example, the importance of this type of real-world evidence. And not only, for example, kind of, understanding the patient journey, let's say, to support discovery, medical, R&D-type use cases, but also she mentioned the forecast. And so, I think what's really unique, is that clinicogenomics, as a data type, and certainly as a type of evidence we can generate, as an industry, has such relevance, across the full, kind of, supply chain, or just all workflows in pharma. So, I'm curious, Ashley, if you can maybe just touch on, from your experience, supporting the pharmaceutical companies that we have the pleasure of interacting with at Optum, or certainly, just in your days as a scientist or in academia. What are the most appropriate roles, for clinicogenomics, across the value chain? Whether it's discovery, medical, R&D, or even commercial.
[Ashley]- Thanks, Eze. Right, I have been in genomics for a long time, over 20 years. And, when I started, it was before the human genome project had been completed. And we thought that when this happened, we would have a cure for everything and we would understand everything. We were very naive, right? An aspirational view, but it was very naive. And, initially, where I saw a lot of the impact of this work, was in biomarker discovery. In discovering new druggable targets, really in that early R&D setting. And what I have seen change over time, is that yes, we're still definitely using clinicogenomic data, in that early R&D space, but also, in a number of other areas as well. So, for example, building out a profile, of a candidate for a clinical trial. Thinking about clinical trials, thinking about digital control arms. How do we use RWD and RWE, in clinical trials? Thinking about things like, market access, or even working with biopharma partners, to understand who's prescribing their medications. These targeted therapies, who else was potentially eligible, to receive a targeted therapy but didn't, and why didn't they receive it? Is it that they're not receiving the right types of genetic testing? Is there provider education, that could help this situation? And really thinking about how the molecular testing, can compliment the biopharma products.
[Eze] - Thank you so much, Ashley. Great insight. Kind of, redirecting the dialogue a bit, to Naveen. And so, what really struck me, in your response, with just your general commentary about this particular topic, is essentially, one of the major, if you will, benefits of the exhaust data, the real-world data, associated with the blood biopsy. And that is the sequential analysis, or the longitudinal analysis, that it can empower, versus traditional IHC, of course, you would argue. But, with that being said, I was, a few former team members last night, relevant to what the industry could argue is the flagship clinicogenomics asset. And one of the major pain points they expressed to me, was the, kind of, the lack of clarity, within that particular platform, in terms of being able to gauge tumor response. And so, how can that sequential data, Naveen, how can that sequential data, that is derived from, let's say, a blood biopsy, how can that support additional insights? For example, on very unique endpoints, such as tumor response, drug response, and the like.
[Naveen] - Yeah, it's something that, we as Guardant, have spent a lot of time thinking about, internally here. And you know, there are, you know, smarter folks than I am, working on this problem. But this concept of molecular response, which is to me, really cool and really exciting, to see really, kind of, have some traction here. But the idea is that, you can analyze genomics, from, you know, a blood sample and look at things like, the overall burden of the circulating tumor DNA. And within that, you know, fraction, the relative fraction of certain variants, within that circulating tumor DNA, to sort of, assess molecular response and how patients are responding or hopefully, you know, hopefully responding, but potentially not, to certain therapies. And so, this is something that, you know, Friends of Cancer Research, for example, has taken a really strong leadership position on, in terms of bringing this into clinical care and you know, demonstrating that utility. And it's something, obviously, that, you know, near and dear to our hearts, here at Guardant. And yeah, I think, the beauty of a, you know, clinicogenomic data set, right? Is that you have this, sort of, validation, you know, ready made, in the sense that, you can look at the molecular changes, in a patient's tumor and see how that, might or might not, in some cases, correlate and be concordant with what you see, from a clinical perspective. And we've already seen, there are cases where, you know, identifying or you have the ability to identify, you know, progression, potentially, earlier than you would by looking at anatomical scans looking, you know, at the genomics of that tumor. Some really great work, more recently, in breast cancer, around that topic. So yeah, that to me is, you know, kind of the, you know, one of those high value use cases, of liquid biopsy that, I think, can really be, sort of, born out through analyzing real-world evidence.
[Ashley] - Naveen, I'm gonna jump in with a question, that's really, for my own edification. You were talking about, you know, looking at a molecular response. Do you think that, moving forward, this will be used, in partnership with imaging for recurrence monitoring, or more than imaging for recurrence monitoring? How do you see that change? [Naveen]- Yeah, it's a great question and I think it largely depends on the clinical context, right? So, there are cases in which, you know, getting a scan is not a big deal. There are cases where getting a blood draw, is significantly earlier- Or easier and, you know, sort of easier to execute. And where I think the, sort of, ultimate outcome lies, is if we can demonstrate, and I guess, you know, that's we, being Guardant in this case, in the field. That like guess like, as I mentioned, you can identify that signal earlier and thus patients are better off, you know, being switched to new therapy potentially, you know, before you see a big mass in a scan, right? And that, you know, honestly is an open question, but, you know, early data, again, indicates that we might be onto something here.
[Eze] - Thank you so much. So, I'm just taking my screen off of sharing mode, just for a second. 'Cause I think we had a bit of a tech issue here and so I'm just gonna reshare my screen and move on to the next topic, for the panelists. This is a topic, that I find incredibly exciting. I referenced it just a few moments ago, but one of the key reasons why we led, with the FDA guidance, from earlier this year, is because in 2021, two-thirds, specifically, 33 out of the 50 drugs approved by the FDA, had some type of reference information in their submission and the regulatory approval process, that included genomic insights. And so, wanted to ask our panelists, to really, kind of, share their perspectives on how the- Or what the implications are, for our Life Sciences ecosystem, given that genomic data has been directly implicated, in the approval process. As most recently, in the calendar year. And so can we begin with Nuray, just your perspective on-
[Nuray] - Sure. Definitely, Eze. I think, I mean, I think this goes to the excitement, that we have been talking about. I mean, right, I mean more and more different types of data, and Ashley touched on it a lot, right? Like, now the quality of the data, demand for data, is increasing, the quality of the data is increasing. So, they are actually helping each other. This is great news because this actually means, now, in the future for drug development, now genomics data is gonna become more of a norm, rather than, you know, as a good add on, or whether if we can get it, then we will get it, right? Like, so it now becomes a lot more the norm and that will actually flow this data through the continuum of the cycle you mentioned, right? Like, the drug discovery. And I, you know, I'm more on the commercial side, right? So, I've been hearing the genomics data, for a while, but now, I mean this year, you know, as we are partnering, doing something, even in this space, phase two, you know, basically phase two and on, now it's becoming a, you know, possibility. Why? Because initially it was like, so rare, that we only thought about it, okay, maybe pre-drug discovery or during drug discovery. And I think that has a huge impact now, to think about this, in the whole continuum of the work stream. There's still, you know, things to be done, right? I mean, at least from those folks, who have been in the genomic space, it's still a very huge investment for companies. This is a very still, expensive data. I don't only mean expensive for pricing purposes, but the resourcing, the tech component that's needed, you know, right? So, it is a pretty, you know, still, you know, hard to use for some of the technical, from the technical aspects. When you think about pharma industry, or the Life Sciences industry, and we still have a way to go in that setup. But in my opinion, you know, for all the data geeks out there like us, I think this is great because now, we really are talking data, right? Like, it's not only the claims data we're talking about, it's not only the, you know, nurse recording data, from a clinical trial, you know, with their hand recordings and then entering it to a system and then we're measuring it. This, actually, is more cohesive, look to the humans, the patients, not only the claims, the payers, as we said, right, doctors. So, you actually allow, with the data, you allow a lot more partnerships, about the patient, as well. The lab companies, the doctors, the, you know, the payers. And so, to me, the more, you know, structured data there is, and I consider genomics, a little bit more structured, in that setup, right? So, the more data that it's easier to really collaborate, as well, you know, from a patient to physician, than to payer, to the pharma companies. So, to me, this is actually super exciting and I can't wait for more to come. But I think it's getting more and more to be the norm, rather than the, you know, good-to-haves or the, you know, oh great, this is like a great news because it's just very unique, right? So, now it's more the norm. And I think that's actually great to know.
[Ashley] - Something that's so exciting about this, I think, is that it looks at the underlying pathways, with drug targets, right? So, for, in cancer, for a long time, we thought about cancer as a site specific disease, right? It's breast or it's colorectal, but really, more and more now, we're thinking about it from a pathway perspective. And this allows us to be much more precise. With these drugs that were approved last year, we haven't really talked outside of oncology, but these were really pan-therapeutic. And so, not only are we seeing clinicogenomics, used in oncology, where we saw early adoption of precision medicine, but also outside of oncology. And if we think about specific therapeutic areas, like, autoimmune and inflammatory conditions, we know, from a science or a systems biology approach, that there's a lot of overlap in the pathways that are involved. And so, this, potentially, could also up, new ways to use existing medications, that I think is exciting. And we do, we have a question from the audience, that I'm going to bring up here. Nuray, I'm gonna put you on the spot a little bit. Feel free to defer, but the question is, 'How important is clinicogenomics, to the field of tissue agnostic drug development?' And I think I touched on that a little bit. Now, I know you're not clairvoyant, but the next part of the question is, 'Do you think that a clinicogenomic data package, will become the standard? Rather than, sort of, an exception, in the regulatory approval process?' Do you have any thoughts on that?
[Nuray] - Yeah. No, I think that's exactly, I think, great question. And totally to the point. That's kind of, I think, what we have been, you know, discussing. First of all, the fact that if data is coming, more and more closer, to the real-world data and evidence, right? The dimensions of the data is accepted and eventually we will have to go to the- When we say data, we're not gonna mean one type, right? We're gonna mean everything that's linked and, which is actually my excitement, right? It's not only even clinicogenomics data, it's the match with the EMR data, match with the claims data. So, now we actually have a much fuller picture, about what happened to this patient, in terms of the genomics aspect. In terms of the, their journey through the health system, in terms of their doctor's perspective, from the EMR system, right? So, to me, that's actually the biggest impact, I really think we will get there. Of course, you know, when and how, is still unknown to me because it's just, the technology needs to move faster and the technology, the talent, you know, all of these things matter, you know, right? So, as we get into this more and more, the talent also will develop, you know, to understand more about the genomics data. Right now, you know, we're kind of, getting there, a little bit, but it's not too far from it, to be standard. That's kind of how I see it. You know, when you mentioned, it's funny, the claims data, and I was a data analyst back then, right? You see a code and you're trying to understand what happens with the lab test, or whether they even ordered the test, and then when did the even doctor got the results and whether the doctor was able to read the results because the results were like very different, non-standard, you know, from there to here, we came a long way. Now we're talking about genomics data, the whole panel, like, I think to me, the moment was actually pretty fast, so it's not, it wasn't slow. So, I definitely... That's kind of my perspective. I think we will get there. Not too slow, but there's still some steps that we need to complete, to make it a standard.
[Ashley] - Oh, absolutely. And it's interesting how the challenges have changed. So, for a long time, granted, I'm biased here with genomics, it was, obtaining the genomics data. It took a long time to sequence a whole genome, and it was really expensive. It was really cost prohibitive. And now we have, you know, everybody's seen the news, right? Sequencing costs have come way down. It's really super quick. And now we're able to also generate, say, RNA-seq insights rapidly and at pretty low costs. And the challenges now have moved more towards the tools to analyze and understand and interpret, those types of data.
[Naveen] - Yeah, and building on that a little bit, I like the last part of that question. Which is, sort of, how this is gonna impact cost. And I think this is a really important thing to consider, is that, introducing a genomics element, into a drug development program, doesn't necessarily mean you're gonna triple the cost, right? And in my experience, and kind of what we've seen, supporting a lot of clinical trials, is that trials with better biomarker strategies, tend to be better financial enterprises, as well. Meaning, you're not doing a thousand patient study, where your risk of not working, given your biomarker unselected, is really high. The other thing is, you know, with, you know, going into a trial, with a data package in hand, it's sort of the, measure twice cut once concept, right? So, you can understand a lot about your intent to treat population, just by looking at the data. So, you know, how are they being treated today? You know, what else is going on genomically, that might be, you know, something to consider, you know, in your therapeutic and development strategy. So, there are all these things you can do with data, you know, in hand and with, you know, a very thoughtful biomarker strategy, including things like, digital control arms, right? That dramatically reduce the cost of, you know, a big, you know, development program.
[Ashley] - And not to mention, the patient cost, right? If you get the right therapy the first time, it's much better for everybody, when it comes to healthcare utilization, when it comes to patient response. We had a question from the audience, that I think is relevant here. And it was, 'are patients more accepting of advice that treatments are unlikely to work?' And I've done a bit of research in this space. We ran some studies, where we looked exactly at this. And what we found is that, when there is genomic data, that a provider can discuss with the patient, we found that there was more acceptance because it was objective information. But it also helps strengthen that relationship, so that other alternatives could be found.
[Nuray] - And I think, in my opinion, also, like, the patients' perspectives are changing, right? You know, a little bit more, being safe, you know? Like chemo, for example, in the oncology space, and my team does a lot of market research, as well. Like, chemo was like, an accepted endurance, for a lot of the oncology patients, right? The minute they get the diagnosis, they were like, yeah, okay, fine. Now I'm ready for chemo. And now, patients are questioning that. Do we really need it? What does it do, right? Like, do I really need to go through this? Like, I think, to me, this questioning as well, like the, you know, expecting better life quality as well, not only the treatment. Okay, I'm gonna endure everything you tell me, doctor-type attitude, I think, as you mentioned, right? Is also changing it a little bit, right? So, if there's no benefit, why should we do this, right? So, all of these questioning as well, in my opinion, is changing. And that's actually why, now data is becoming much more important, because it's objective, everybody can access to it, everybody can see, and everybody talk on top. Versus the knowledge the HCP has, you know, through their background, the patient, the knowledge the patient has about their life, the knowledge the payer has, and they're all different, it's very hard to really come to a conclusive state. But then, now it's actually a much more objective and shared, you know, shared story, I would say, as data, you know, being in the central point.
[Eze] - So, great conversation from the panel. I just want to- I can't help but think about, a few, or one specific word, that kept resonating, during the rise initial commentary. Which was the reference to structured. Unstructured data, which is, for example, associated with traditional types of RWD like claims. But curious, if the panel can comment on the role, of not only integrating, for example, EHR and claims with external assets, such as blood biopsy data from entities like Guardant. But what about the role of unstructured insights, moving forward, in terms of how you can develop a more robust clinicogenomics assets to, for example, communicate your value, with regulatory authorities, like the FDA, or even insurers, such as, those that might cover the patients, that are ultimately eligible for your therapy. Ashley, any thoughts there?
[Ashley] - Well, that's really- There's a lot of meat in the unstructured data, right? We all wanna see the notes. The challenge, historically, has been that, these contain personal health information. And so, there have been a number of efforts. I know, certainly, our group at Optum has, put forth a lot of effort, in developing NLP, that can pull specific data elements out of the notes. And then restructure them, so they're structured data, that are easy to query. But the challenge has always been in the re-identification risk.
[Nuray] - And one thing that I wanna add on, I think this was a great question Eze, as I actually mentioned, right, we're all interested in this. But then, we also have to accept the subjectivity of this data, right? Like, so when you go to one healthcare system, versus another healthcare system, the way that it's - I know we always consider science, being very objective - but the way that this actually, unstructured data, being very subjective, is also very hard, makes it very hard to analyze. The way that you go to community centers and community practices, versus academic centers, academic practices, west coast, versus east coast. I mean, you would be very surprised, like, how, culturally, the way that they're reported, the way that they're actually referred, is so different. So, it actually makes it very hard for someone, to really, make it objective, across the nation, for U.S. And I'm not even gonna mention, as you know, my role is global and Europe, on its own, is its own world. So, I think that has been the challenge, as well. I mean, I think it's a good challenge to have. We're all excited, we're all, you know, ready for challenges. So, we are still working on it, but I think the subjectivity of it, is also, to me, is actually the hardest piece to solve for.
[Naveen] - Yeah. And it's funny, the first word that came to mind, was context, right? And having context that you can apply because I think of anytime we are looking at our data and we're kind of stumped and can't figure out what's going on. You know, we check with our med affairs and it's always some quirky thing, about the, you know, practice group or physician, or something that, ultimately, is what explains what we're seeing. So, I think, you know, that's something that's really important to keep in mind, is having, you know, in addition to the data, right? And having high quality data and data scientists and everything else, having, you know, that clinical, medical, scientific context, for what you're looking at, is invaluable.
[Eze] - Well, thank you again to our panelists. Great conversation, but also want to thank the audience because there've been, really, just an influx of questions, that have been entering, kind of, the portal here, that are guiding the conversation, as of recent. And so, please continue to ask away. And if we cannot address your questions live, we'll certainly take the opportunity to follow up and provide guidance and commentary, where we're relevant on your given inquiry. And so, thank you again to the audience, for being so active in participating. And so, switching gears to, unfortunately, our second to last topic for today's conversation. And this particular topic, really does resonate, hopefully, with Nuray and her outstanding team at Novartis. Because we wanted to take, just a few minutes here, and talk about a very unique scenario, in the real world, for a combination of target therapies, within the oncology marketplace. And, specifically, a combination that targets the BRAF-mutated population, or the BRAF-mutations, in the specific non-small cell lung cancer populations, that have a particular variation in their genomic profile. And so, Ashley, I guess, what do you find compelling, in terms of, the results that have led to the ultimate approval of dabrafenib, or trametinib, i.e. Taf-Mek in not only non-small cell, but really, across the entire patient population, with the implicated BRAF tumor?
[Ashley] - Yes. So, what's so interesting about this, is that this particular BRAF-mutation, is quite rare, in non-small cell lung cancer. And so, it's really difficult to obtain the participation of a sufficient number of individuals, in order to see, to really judge treatment effectiveness, or response, or progression. And so, including, this group included real-world data and compared that to clinical trial results. And they found, really, a similarity in the patient outcomes and they were able to, more greatly increase their total sample size, by including real-world data, along with clinical trial results. And this allowed them to measure the efficacy of this regimen, in this particular patient population. I think this is increasingly important, as we talk about rare variants, but also rare disease. If we're enrolling in traditional clinical trials, it can be really hard to obtain the participation of enough individuals, so that we have statistical significance. And using real-world data, allows us another avenue, to increase the sample size and to increase the reach, of some of these studies.
[Nuray] - And, just to add on, actually, I think you touched on a really important point, where, you know, precision medicine has been slow and everybody, kind of, complains about the pharma companies who wants to launch these billion dollar drugs, but I actually don't think that's actually the situation. The situation is, when you're trying to make sure the trial, you know, involves this many numbers and some of the trials for rare diseases, I know, couldn't even meet their endpoints trial. And you can imagine the cost, exponentially growing, just because of this patient, not being able to get to the trial or you are not able to find them, you know, basically. So, that's actually, to me, is actually the biggest aspect, right? Like, so, at the end, I don't think it's only the financial aspect of it, it is really more, the return on the investment, if you're not able to invest and then get your return. Because even though when you invest and you wanna really create a cure or a treatment for some of the rare forms of diseases, but you're not gonna be able to prove it, you're not gonna be able to recruit for the trial. So, I think it prevents some of the companies to get in. I mean, obviously, I'm not talking, specifically, about Novartis But in the whole pharma world, I think that, kind of, prevents a lot of the drug companies or the biotech companies, even small companies, to get into these, some of these rare diseases. Eventually, this will also, bring down the cost of those trials, expedite some of the trial recruitment. To me, that's actually great news for everyone. Not only for the patients, but the pharma companies, the doctors, so that this actually will enhance a lot. I think that, in general, the treatment opportunities for everyone.
[Ashley] - Yes, I think you touched on a really important point, which was access, right? When we think about these traditional clinical trials, there are specific locations, where these trials are held. But this allows us to look at evidence for individuals, throughout the world, or certainly throughout the country, without that regional bias, that might otherwise exist.
[Naveen] - Yeah, and the other thing I would throw in here is the ethics aspect of this. And so, I think, you know, someone said this, we have enough data on patients, who've been treated with chemotherapy, right? So, having a control arm, where you have a hundred patients, you know, who have been biomarker selected, potentially, and going on untargeted chemotherapy, I mean, feels, borderline, unethical. And so, you know, using external control arms and real-world evidence, you know, is a way of, you know, in my opinion, you know, really making sure we're maximizing patient benefit.
[Eze] - Thank you so much. And I think that is a great springboard, to the last topic that we'll be touching on today, formally, during today's presentation. And that topic is focused on, not just clinicogenomics, but, if you will, other types of -omics, that we can leverage, as an industry, to really drive innovation and push healthcare forward. And so, here are just a few different types of -omics data, that we are consistently evaluating, here at Optum Life Sciences, and I wanted to just really pass the ball to Ashley, to comment on maybe how unique, or what unique benefits each of these types of -omics data, can bring to the mix, if you will, and, likewise, where we are, in terms of, the level of integration and utilization, at this point, in the industry's standard workflows.
[Ashley] - Thanks, Eze. Yes. This is so exciting, because it's the progression of -omics, right? Now, we're not just talking about, those single genomic variants, we're talking about exomes or we're talking about genomes and now we're talking about the transcriptome and we're talking about epigenetics, even the microbiome, right? And how these all work together, to show us what's truly happening, in a real-life human, in the real world, right? We don't exist in a vacuum and so this helps us understand, that entire patient picture. I think the challenges there, are in the quality of the data, the accessibility of the testing, and also in the utility. So, is it all important, all the time, right? And that's a question that, I think we will continue to work with, as this continues to advance.
[Eze] - Thank you so much, Ashley. And so, Naveen, given that Guardant is on the front line, of really just innovating, in term in terms of -omics data. Any perspectives from the broader, kind of, industry expertise that you possess, that you can share with the broader audience, as well as the panelists, on the expansion and utilization of other -omics data?
[Naveen] - Yeah, absolutely. I think, you know, a lot of this work, as I mentioned, it's hard, right? And so, having more data, is generally better, but I completely agree with Ashley's comment that, that's not always the case. And making sure that the data is of high quality and, sort of, meets certain quality thresholds and things like that and can be really, you know, placed into context, it is gonna be really, really important. So, data for its own sake, you know, is not always gonna be the answer. I know that's sort of antithetical to say, in this setting, but I think it's worth noting. On a more, kind of, positive note, one of the things I find, I'm sort of super excited about, is, you know, the increase in multiomic data sets. So, one of the things you know, I've done, historically, is, you know, for example, look at the genomics and transcriptomics and, sort of, do some really interesting core level analysis there. And I think, you know, we can start, sort of, layering the different, you know, data modalities, you know, you tend to see even more than you could, just via one. You know, Guardant, is sort of, moving the direction of really exploring the methylome. So, we just talked, we released publicly, or release of the, sort of, smart liquid biopsy platform, that captures some methylomic data. And I'm super excited, as we, you know, as we transition to that, you know, portion of our, you know, journey here, of being able to actually incorporate that data into some of the work we're doing, with our, you know, external partners, as well as internally here, to really understand, you know, not just the genomics, which in many cases, is, you know, relatively cut and dry, right? But the methylome and how that, you know, changes. And, 'cause that is, you know, obviously, as we all know, highly dynamic. So, you know, lots more to come there, but that's, you know, what, personally, I'm most excited to see, over the next few years here.
[Eze] - Thank you so much Naveen. Nuray, any comments from your side, on just the relevance of additional -omics data, to the Life Sciences, since you're on the ground, leveraging these unique data types, to support various commercial, as well as R&D workflows?
[Nuray] - Yeah, no, I mean, I think, Ashley gave a great summary, you know, basically. And we are all learning and tackling these pieces, as it comes through. You can imagine, right? We've started with prescription data, in my old days. So, you can go from here, now to multiple -omics data, is actually super exciting to, basically, live through. You know, genomics, we're just, kind of, putting our feet into, you know, kind of, we're trying to use it and I think, once it becomes the norm, the other -omics data will also follow easily. That's, kind of how I see it. But in the meantime, you know, I think the critical piece will be, and again, this has a huge impact, on the data science talent, in my opinion. The larger the data, the more talent that's needed, to really summarize this and understand what this means. I've actually observed this, in the marketing space, in the pharma, right? We used to have prescription data and then market research data. You just had two pieces of data. Now we have so many different databases and so many different data sources that's coming through. Now the critical aspect becomes, not the data utilization, is that now after you use all this data, what this means, for your marketer, or your salesforce, or your medical colleagues, right? What this means. I feel like this is also, like, this is gonna be huge for the Life Sciences, in terms of, what will this mean for the doctor, the health institution, for the patient, right? When you bring all of this data, to me, that's also becoming a very important aspect of, as the data expansion occurs, which is awesome, which is great, but then there's actually that, you know, there's more talent that's needed now, who can actually connect these data sets and define what it means. To me, that's definitely gonna be the next round, that we will be talking about, after all of this data sets becomes, more the norm.
[Eze] - Awesome. Completely agree, Nuray, great response. Just to quickly interject, before maybe, we have some closing comments, from the panelists. I think that you're spot on. And so, the talent needs to be available, to work with these data assets because we're not talking about, for example, supplementing EHR or claims with genomics data. We're building and adding more data together and integrating appropriately. And so, I would take that one step further and not only, if you will, just address and emphasize, the need for the talents, to actually derive insights from the data, but also the platforms. And so, it's great to talk and articulate, as well as just, you know, have, kind of, fruitful dialogues about these types of various data types. But we need to be able to work with them, realistically, in key platforms, that are not only privacy intensive, but also allow us to, for example, support discovery, support medical colleagues and the like. And so, Ashley, any comments on, maybe, platforms, or the evolution of platforms, that are able to accommodate just, these very large data assets, once we start integrating? Not just the genomics data, but other types of unique assets, such as transcriptomics and the like?
[Ashley] - Sure. So, you're right. We need a place to store all of the data, to link all of the data and to analyze all of the data and we need the proper tools in which to do it. We have seen quite an evolution, in what is available. So, traditionally, everybody had their own analytics platform and you would, you know, write the code yourself and try to make it happen. And now we have seen the rise of some very good platforms, where you have an all in one package, where you can store and where you can analyze.
[Eze] - Thank you so much, Ashley. And Naveen, again, because you are coming from, such a unique perspective, working for one of the premier institutions, when it comes to -omics data. And so, whether it's internally and externally, or externally, in terms of partnerships. Any broader comments, on like, the technology platforms, that are supporting your business and now you see them, either currently being leveraged, or how they're evolving, moving forward, to accommodate, frankly, all of this data?
[Naveen] - Yeah, well, you nailed it, with one of the words you used there, which is partnership. And so, one of the things that we're always, you know, open to, here at Guardant, is clever partnership. So, you know, we have our, sort of, core technology, that we, you know, have spun-up in house. But, you know, in terms of, finding best in breed partners, for both, you know, the data side, as well as technology, to enable all the things that we can and should be doing, you know, we definitely have taken that opportunity, when it's arrised. So, yeah, I think, that's sort of my takeaway here, if you will, is that, you know, we're really creating an ecosystem and you know, finding the right partners, can be just as critical, as, you know, doing things yourself.
[Eze] - Thank you so much. And so, maybe can we just ask each of the panelists, to provide maybe a few closing comments on how, not only you, kind of, foresee the evolution of clinicogenomics, but any, kind of, just unique nuances that are, really relevant to you, at the top of mind, in terms of, how you can leverage this type of asset, moving forward. Can we begin with Ashley, to begin, kind of, the wrap up process here?
[Ashley] - Sure. Thanks, Eze. I think this is a time of great excitement. That's really what I see. There's constant progress. We're moving forward. We're doing this very strategically and very thoughtfully. And I love what Naveen said, about partnership. I think that, we are now in an environment, or in a state, where we can really leverage collaboration, right? So, we can see molecular diagnostics, collaborating with, you know, data and real-world data providers, partnering also with pharma. And I think, together, we can all really be strategic and thoughtful, about how we provide the best treatments for patients.
[Nuray] - I think, what I wanna- Yeah, and what I wanna continue with, I think, is definitely we're gonna move forward. I think that's kind of a conclusion, right? There's, I think, no going back, with this much data. So, I think that's why, to platforms, to talent. I think, the healthcare system, is coming closer, you know, right? I think to me, the big value of the data, as well as, you know, the lab companies, the -omics companies, the pharma, the health institutions. I think, to me, that's why those partnerships, not only within the industry, but now, industry, academia, you know, I see that a lot. I think that's also what's happening, is the more data is coming through, it also is, you know, creating a much better partnership environment, you know, across the board, for Life Sciences and for the patient, which is actually super exciting.
[Naveen] - Yeah. And I guess, my, sort of, closing comment would be, kind of, one of our mantras, here at Guardant, which is, 'fit for purpose.' And so, that's something we always strive to do, is when we apply data towards a given problem, we always start with the same question. Which is, what is the question, right? And that will, kind of, dictate where to take it. So, if I were to, sort of, leave folks with one, maybe, you know, I wouldn't call it wisdom, but word, it would be, fit for purpose.
[Eze] - Thank you so much, to each of our panelists. And thank you again, both the Optum, Novartis, Guardant Health, as well as Endpoints Webinars. Have a great day.
[Naveen] - All right. Thanks everybody.
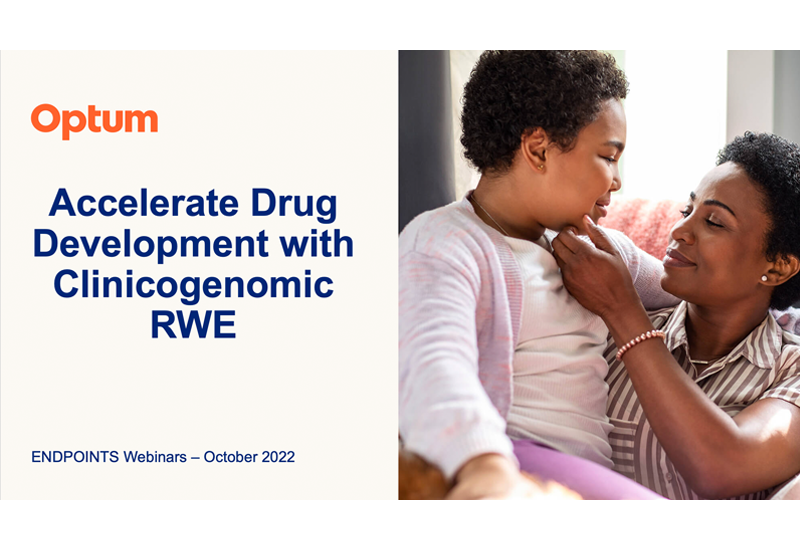
Accelerate drug development with clinicogenomic RWE
Join our panel of experts during this Endpoints News webinar as they explore the ever-increasing importance of clinicogenomic data, highlighting:
- The importance of diverse phenotypic and genotypic profiles
- Improving regulatory approval outcomes
- Identifying new biomarkers for treatment response
- Label expansion by expanding existing treatments to new cohorts or conditions
- Using real-world evidence (RWE) as digital control arms for clinical trials
Our panel
- Dr. Nuray Yurt, Global Head of Artificial Intelligence, Novartis Oncology
- Naveen Kumar, Founder and Head, GuardantINFORM
Eze Abosi, Vice President, New Products, Optum Life Sciences (moderator)
Related healthcare insights
Article
Understand how routine clinical practice impacts information captured in real-world data (RWD).
Video
Optum Life Sciences leaders break down common missteps when using RWD and how to create practical strategies to overcome them. Watch the video from STAT Summit.
Article
Providers collect data about patients every day. But what should they be considering — and doing — to put their clinical data to good use?